Introduction
Artificial intelligence (AI) is increasingly seen as a powerful tool for making everyday tasks easier. Many work processes can be optimized, and new solutions can emerge – but we cannot ignore the importance of data security and the implications of using tools powered by large language models (LLMs) such as ChatGPT. Especially in environments where AI can interact with documents, emails, and calendars, it’s critical to ensure that usage is both legal and responsible.
We’ve encountered many managers and IT professionals who are excited about AI’s potential, but at the same time are concerned about violating GDPR. They worry whether AI tools might leak confidential information or amplify biases in data. That’s why we’ve compiled this comprehensive guide – to help you navigate a landscape where innovation and privacy must go hand in hand. This text places a special focus on data protection and the use of LLMs, helping you understand how these technologies can coexist with strong privacy safeguards.
This article dives deep into data security, ethical AI, and regulations like GDPR. The goal is to provide a holistic understanding of how to protect privacy, respect legal obligations, and still harness the power of AI. Whether you’re a project manager at a mid-sized company, an IT lead preparing your systems for AI, or a marketing professional dreaming of personalized email campaigns – there’s something here for you.
The history of AI and its connection to data security
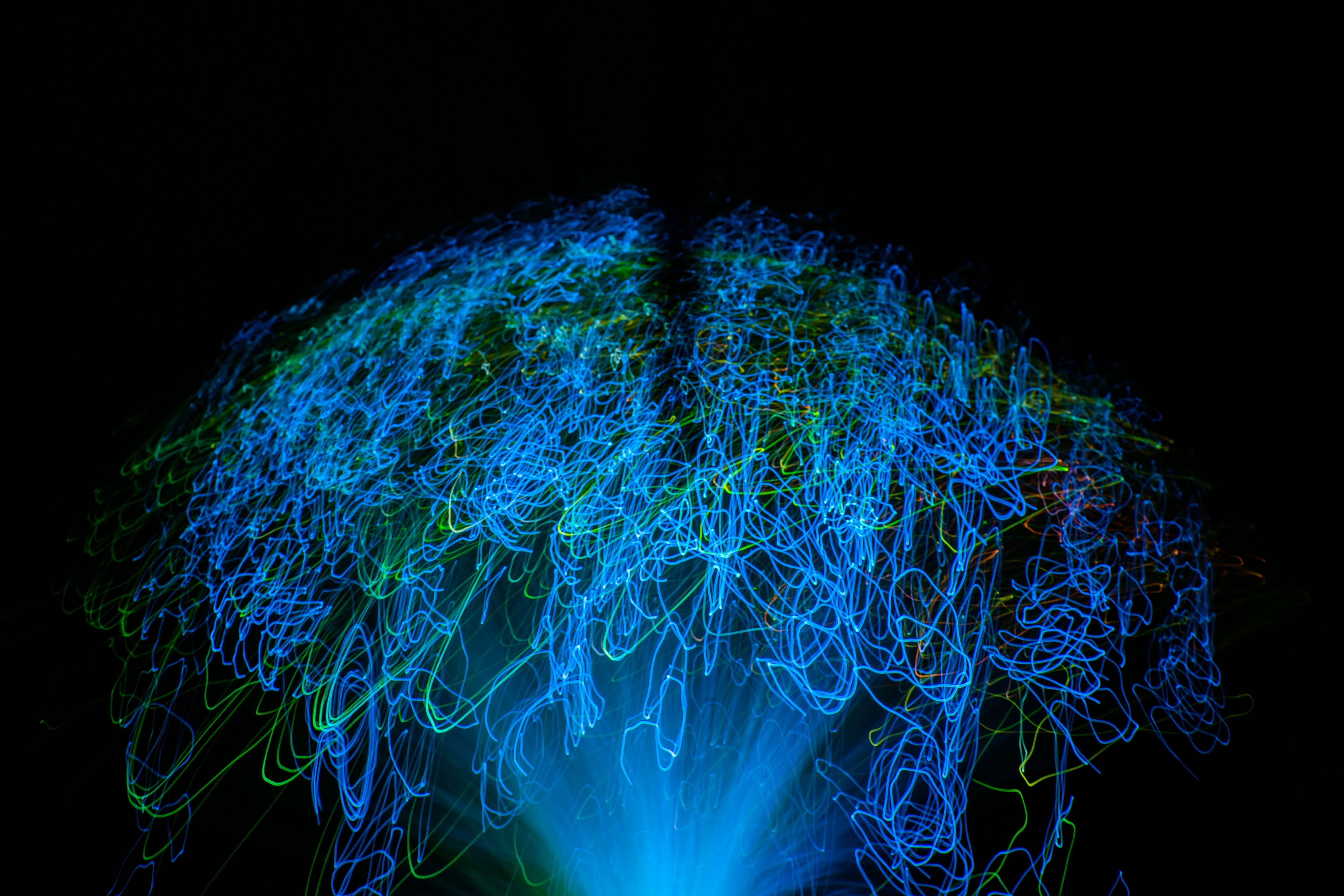
AI isn’t new. As far back as the 1950s, researchers attempted to create computers that could “think.” However, hardware limitations held them back. Things changed when computing power became cheap, and data volumes exploded. Suddenly, models could be trained on massive datasets – and computers could predict or generate text with surprising quality.
With the rise of AI came increased concern over privacy. Companies have been caught collecting users' data without their knowledge. Some used this data for profiling, targeting ads, or political campaigns. The Cambridge Analytica scandal showed how tech advancement can go awry if ethical considerations are ignored.
With the GDPR, the EU created a regulation that puts citizens at the center. People gained rights to access, delete, and understand the purpose of data processing. At the same time, companies faced strict security and accountability demands. This was a game-changer – and the question became: is AI even compatible with GDPR?
From early AI to data security and LLMs
Today, AI is part of everyday life, from streaming recommendations to writing emails. Tools like ChatGPT, Google Gemini, or Microsoft Copilot are being integrated into workplaces as intelligent assistants. They can generate text drafts, analyze data, and summarize meeting notes. But to do this, they need access to your documents, calendars, and conversations – which brings data security and compliance to the forefront.
Since the introduction of GDPR, data protection authorities in the EU have actively enforced the law. Companies have faced significant fines for mishandling data. Meanwhile, the EU is preparing a new regulation – the AI Act – that classifies AI systems by risk levels and sets new standards. While the U.S. takes a more fragmented approach, the EU is moving forward with centralized, strict rules. The bottom line: using AI requires a strategic approach.
We’ve noticed that Danish companies often ask questions like: “Can we use ChatGPT if we handle sensitive personal data? What are the risks? Is it compliant to let AI suggest email content?” Our answer: AI can absolutely be used – but it must be done thoughtfully.
With that context, data security and LLMs will be the central focus of the rest of this guide.
Technical Understanding of AI: Focus on data protection
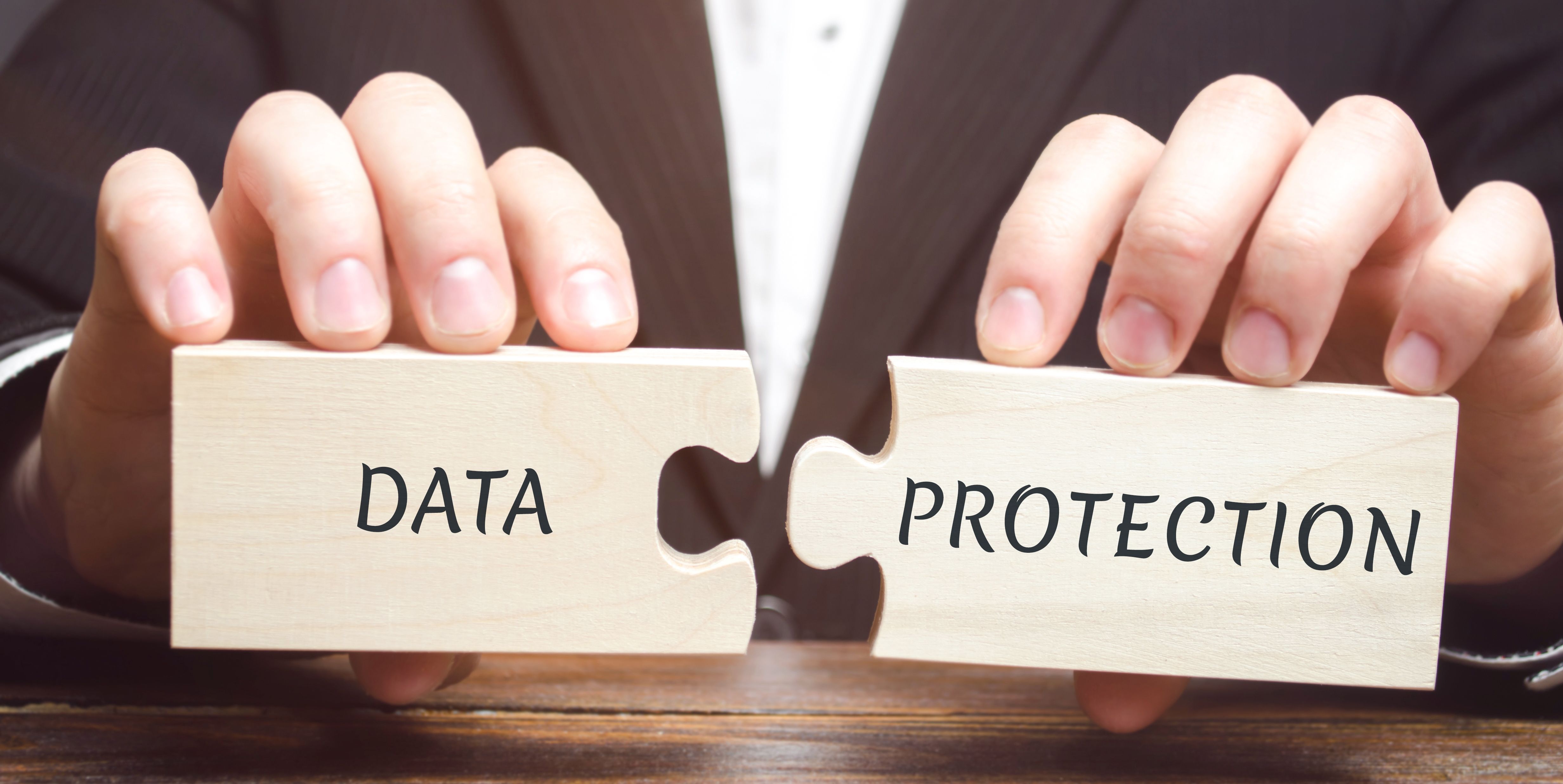
What is a large language model (LLM)?
An LLM is trained on vast amounts of text and learns to predict the next word based on context. When integrated into workplace tools, it doesn’t just offer general knowledge – it can also access your documents (with permission). This enables personalized responses, but also introduces new vulnerabilities – a capability already seen in tools like ChatGPT and Microsoft Copilot.
Security measures
To prevent LLMs from unintentionally sharing data, encryption and role-based access controls are used. In short: if a user doesn't have permission to access a file, neither does the AI. This is part of a “zero trust” approach – no access unless explicitly authorized.
Interaction logging is also critical. Logs can show who asked what, and what data the AI accessed – vital information if the Data Protection Authority ever audits your organization.
Data minimization
GDPR emphasizes collecting only the data you truly need. AI models often benefit from large datasets, but that increases risk. Ask yourself: do we really need the AI to access emails from the past 10 years – or will the last two months suffice? The less data involved, the lower the risk.
Ethical principles for AI: Why they matter
It might be tempting to say, “Just follow the law, and everything’s fine.” But laws are often just the minimum standard. Ethics is about doing what's right – even when the law doesn’t spell it out in detail. Common ethical AI principles include fairness, transparency, robustness, and accountability.
Fairness
If AI reinforces existing biases (e.g., denying loans or job offers to specific groups), it contributes to injustice. Companies must actively test and correct their models for bias – by analyzing output across gender, ethnicity, etc.
Transparency
People impacted by AI decisions deserve explanations – especially in hiring or credit decisions. Everyone interacting with an AI should know that they’re talking to a machine, not a human colleague.
Robustness
AI must be resistant to errors or manipulation. Imagine a user feeding the AI false data to force a specific outcome. Systems should detect and log such attempts.
Accountability
Data security and LLMs are key examples here. If something goes wrong, who is responsible? It’s not acceptable to blame “the AI.” Humans develop, configure, and deploy these systems. Organizations must assign responsibility for oversight, corrections, and deactivation if needed.
Overview of GDPR and the EU AI Act
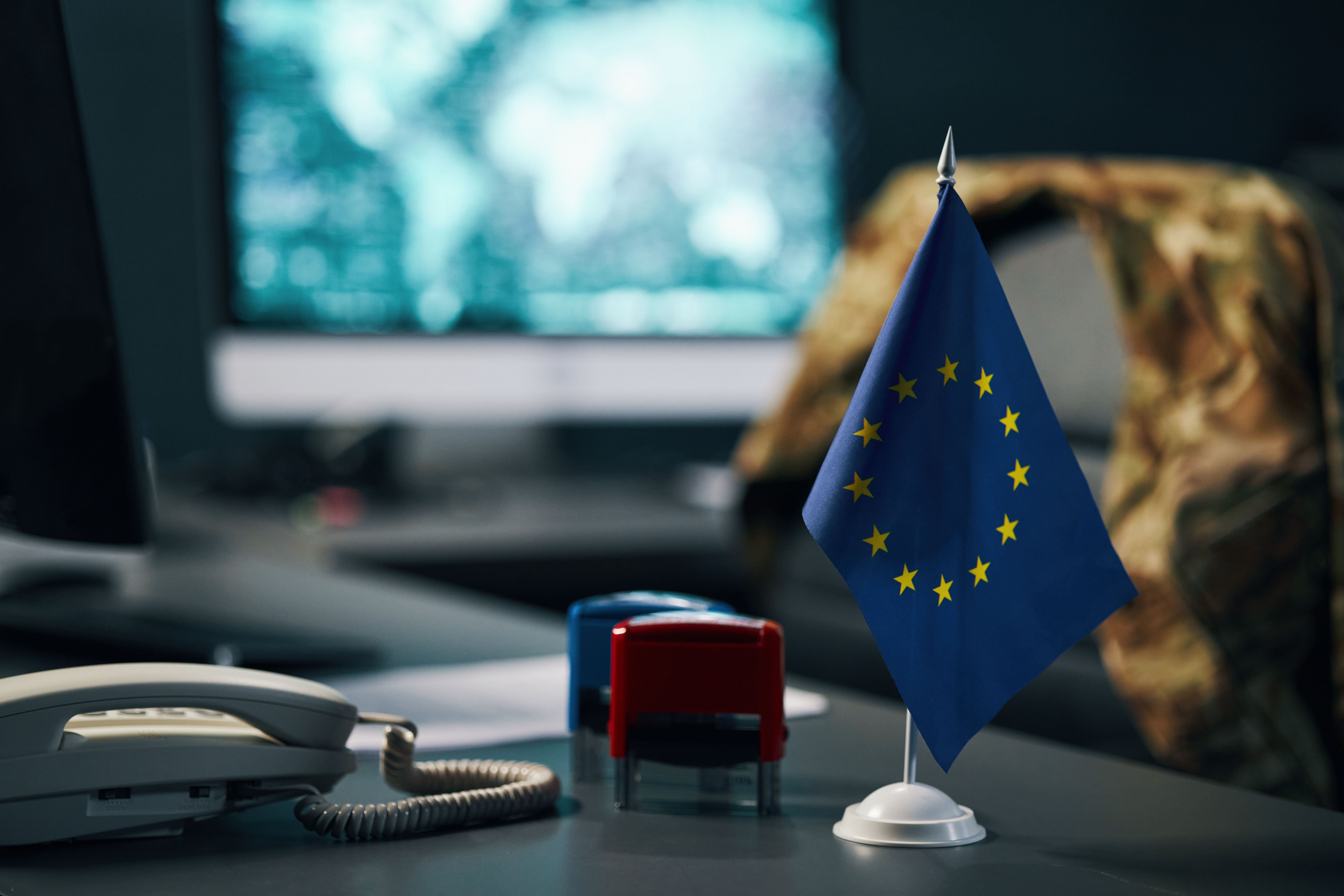
GDPR
GDPR requires a legal basis for using personal data. Consent, legitimate interest, or another lawful reason must exist. Transparency is also required – users must know how their data is used. Key principles include data minimization, security, and purpose limitation.
AI Act
The upcoming AI Act classifies systems into four risk categories:
- Unacceptable risk: E.g., mass surveillance or social scoring – banned.
- High risk: E.g., hiring decisions or critical infrastructure – strict requirements on transparency, robustness, and documentation.
- Limited risk: E.g., chatbots – must inform users they’re interacting with a machine.
- Minimal risk: E.g., AI in games or spam filters – few requirements.
The AI Act complements GDPR by focusing on potential harm from AI systems, even when personal data isn’t used.
Practical example: Using LLMs/Microsoft Copilot in the workplace
Copilot can assist with everyday tasks in office environments. For example, it can read email threads and summarize them, or generate documents from short prompts. This saves time – but the system must access your data to be helpful.
Key Points:
- The model works on top of your existing enterprise data.
- Users must have access rights for the AI to reference certain content.
- Providers (e.g., OpenAI or Microsoft) claim user data is not used to train general models.
- Encryption and ISO standards are implemented for security.
Still, organizations – from large corporations to small businesses – need to establish internal policies. For example: Should HR use AI to summarize employee files? Should confidential documents be excluded from AI access?
Data handling in practice
Controlling access and avoiding sensitive data in prompts is essential. How can you prevent the AI from revealing a social security number in a response? Here are some measures:
- DLP (Data Loss Prevention): Set rules that detect and block sensitive data sharing.
- User Training: Educate staff not to feed sensitive details into prompts.
- Document Labeling: Mark certain files as confidential to restrict AI access.
- Logging and Audits: Track who accessed what, and when.
These steps help ensure AI remains a tool – not a risk.
Implementing responsible AI in companies
Based on our experience, here are some practical steps many overlook:
- Create an AI policy: Define what AI can and cannot be used for.
- Leadership buy-in: AI and security aren’t just IT issues – they require leadership attention.
- Cross-functional team: Combine IT, legal, HR, and communication to define guidelines and training.
- Privacy by design: Factor in privacy from day one.
- Bias testing: Use test datasets to check for unjustified discrepancies.
- Plan B: Have a response plan for AI failures or customer complaints.
- Continuous evaluation: Laws change, data evolves – keep policies up to date.
Organizational strategies for AI compliance
You can embrace AI while maintaining a “compliance-first” attitude by creating structure:
- Clear governance: An AI committee to approve projects and ensure DPIAs are done.
- Standard processes: A checklist before deploying any new AI tool.
- Skills development: Your security team should understand AI risks, and business teams should understand GDPR.
- Defined accountability: Assign someone who can halt AI projects if necessary.
- Consent and communication: Inform users when AI is in use and allow them to opt out where appropriate.
It might seem bureaucratic, but it helps avoid complaints, privacy breaches, and supports a healthy AI culture.
Innovation vs. data protection: The classic dilemma
Some argue GDPR stifles innovation. But GDPR is meant to ensure tech doesn’t spiral out of control. With a strong privacy-by-design model, AI can be developed faster and more responsibly.
Common conflicts:
- Businesses want to collect more data to refine AI. GDPR says: only what’s necessary.
- Pilot projects need speed. DPIAs take time. Consider a “mini-DPIA” to spot early risks.
- Leadership pushes for cloud solutions. IT warns of legal risks. Dialogue is key – technical solutions often exist.
Data security and LLMs – Room for both success and failure
Failure:
A large company launched an AI chatbot for customer service. They forgot to limit data access – so the bot could access the entire CRM system. Users asked sensitive questions, and the bot answered freely. It was a major failure. The bot was shut down, and the company was sanctioned.
Success:
A small business implemented ChatGPT-like tools in a limited scope, accessing only non-sensitive folders. They trained employees to write safe prompts and even added AI usage policies to their HR manual. After 6 months: fewer data issues, high time savings, and a thumbs-up from regulators.
Future outlook: Data security and LLMs will evolve together
In the future, data protection and LLMs will continue to evolve side by side. With cloud computing and 5G, real-time solutions will scale up. EU’s AI Act will shape the landscape. Companies must prepare a robust AI strategy – covering data security, ethics, and governance.
Conclusion and reflection
Data security, ethics, and GDPR in relation to AI are not a “quick fix.” They require a holistic effort where people, technology, and policies work together. We've seen that organizations that take these matters seriously from the outset tend to achieve better outcomes with AI. They experience fewer data incidents, less employee resistance, and greater customer trust.
At the same time, the use of AI presents a unique opportunity to boost productivity. It can save time on repetitive tasks, help generate better documents, and enable smarter analysis. But it’s important to remember that AI is a tool. The responsibility for decisions should remain with people, and data security must be an integrated part of everyday workflows.
To sum up:
- Follow GDPR principles (lawfulness, data minimization, transparency).
- Ensure fairness and transparency in your AI practices.
- Review how AI tools are configured so that users don’t see data they shouldn’t.
- Avoid letting data leave “secure” environments unless permission is granted.
- Foster an internal AI culture that embraces both innovation and privacy protection.
We believe that this very balance – between technical foresight and ethical care – will define future success with AI. And if your organization stands on a solid compliance foundation, it becomes much easier to scale new AI projects. You avoid legal concerns and demonstrate to customers and partners that you’re handling the technology responsibly.